10 Steps to your next Data Migration
- aliciangraham11
- Jan 25, 2024
- 7 min read
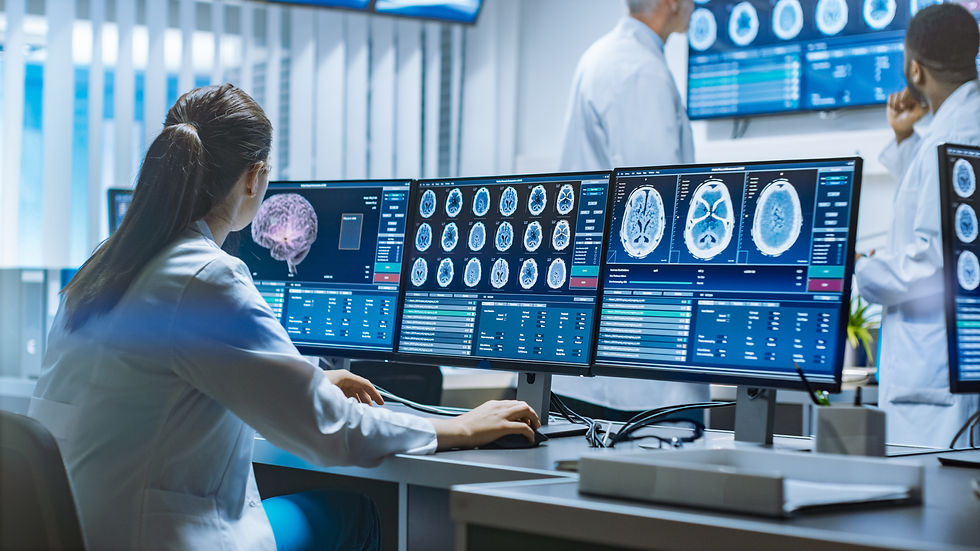
Struggling with what to do? You've been asked to migrate this data - what does that even mean? Data being such a broad term is very flexible and connected if you think about it.
I see data like neurons firing in the body. They are a transmitter of energy (or profit) from one being to another. So how do you wrangle a neuron?
It starts with a list!
Most people I've interacted with like using Excel. Now, I'm not sure if that's the #Xennial in me, or the fact that I've been playing with Excel since I was a teenager or I'm stuck in this adolescence stage (ask me about my Lego passion!).
It could be a little more agile like a Jira project (Jira is an online management tool btw!)
It can also be a list of data centers to decommission
That List needs to be SORTED!!!!
Ok, everyone knows the basics of sorting, but when you're talking about large data sets that you need to make sense of before you migrate to the cloud, prepare to become an expert in data quality.
Sorting also requires prioritization because if you don't know what's in your list, finding the first person who does know something in your list, or you can also start by expanding the details on your list based on your own sense of prioritization.
Last note here, I would make sure you have access to look at the data yourself. The more metadata (for non data geeks, think data about data. For others, think of it like when you go to the grocery store. Some companies will care that you bought groceries, while others will care that you went to Ralph's, Trader Joe's, Piggly Wiggly, Ultimo Mercado, or other grocery #insertretailname here) you have, the better you can understand and prioritize your list/data [see - even the list is now data :)]
Ok, you have a sorted list, now you need to CATEGORIZE
Think of this like adding additional columns in an excel list or adding #hashtags to a video. Is this video a DIY? A stitch with #ERAsTour inspiration?
In the corporate world speak, it's what business vertical uses this data, who benefits from it or uses it, who owns it {THIS IS ONE NO ONE EVER WANTS TO PUT THEIR NAME ON :) ], what data center is it located in?
Wohoo! Categorized list - it's the Grand-kid Round UP! (#encanto anyone???)
As noted above, no one ever wants to own data. Generally I think people can feel the sense of dread that ownership comes with. When your name is on a list, someone, somewhere will eventually ask you about something (#neurospicy ifyyk).
It reminds me of days auditing banks where you had to put a bank on the watch list. Banks were failing in the southeast United States and the #financialsector in Boston (where I was located during the #GreatRecession), #FDIC auditors were cautious of a lot of factors).
Kidding aside, gathering up groups of people who care about data is a challenging task. I typically start with Data Analysts, Data Scientists, Business Systems Analysts, Architects, Database Managers, or really anyone I can get my hands on!
Find the people who are passionate about their data (i.e., like to talk about data a lot. Junior associates are especially interesting to sit beside (#pandemic - it's been a while since I've been "in office") so more realistically join a zoom to achieve something together or have them show you what they care about or are working on.
DoubleBack! (#ZZTop or #BTTF3 fans?)
So imagine you're celebrating! As if in Back to the Future 3, where ZZTop entertains the crowd after breaking up a grandkid squabble. All right - you've done a lot of work to get to this point. It's best to prioritize a creative opportunity to celebrate. For a #neurospicy #mascworkhorse like me, wouldn't you expect I love planning a party? No, well at first, I thought it pointless too! I mean, what are you actually celebrating at this point - a list with names of people - oooh - one's who care or rely on your organization's data?
Believe me, this celebration/team-building activity is crucial. So, find a group activity that can be used to bring these people together. If this can be done in person, I find musical activities (finding a way for people to create sound together) or problem-solving activities like escape rooms are fun, but #becreative! and unique to your team. What personally interests you? If we're talking virtual, fun would be building Lego sets together. I love #Lego so any excuse I can bring it into my life with others is, #bonus!
The closer knit you can get this team, the faster and better your results will be. In my experience, "teams" are best capped at 25. After that, you're in my experience making it difficult to form deep connections. At that point, it's best to think of it as a department/category that needs senior or executive sponsorship.
Be ready to fight the #VAMPIRES!!!
Additional people who ask questions about data after it's been migrated, or after designs have already been approved were are hard demand for my #neurospicy brain to handle. You'd think I'd love the questions, but, once they're long forgotten, they try to come back like a #vampire.
Kidding aside, how quickly people react to changes in data is data to be aware of. LOL, like how I did that there? Created #datainception :)
Anyway, some decision scientists I knew spent quite a bit of time trying to expand a model from 7 years to 10 years. It was really difficult due to the changing regulations around retention. So while data life-spans are being shortened, #Privacy regulatory also provided that certain data is "critical", it will have more business-friendly/profitable protections. The better (i.e., more) documented you are, the longer retention can typically be.
LABELS!! Tapping into your inner Marie Kondo
Labeling in databases, photographs, videos, apps, IoT devices and everything else is how a lot of AI/ML first make their connections. To be successful in migrating, you need to be purposeful, thoughtful, and protective of whatever labels you create.
As soon as a team is larger than 25, it can be hard to keep track of labels. If you find yourself running into that, I suggest creating an Enterprise Data Committee or Data Management Committee, or whatever you call a gathering of people who are data owners and can understand the organizational impact if data is entered wrong [my overly stimulated brain immediately makes sure this project doesn't become (or does if in a compliance sense) goes to #Enron, #metabotcount, #Theranos].
Your goal with this committee is to make as many people see the implications of their choices in advance. For more serious data concerns, this often gets rolled into a Board report of a funnel of tech/other initiatives and if that's the case, in my experience, the migration is likely going to take a while. The faster migrations have consistent reporting and people who want to know what is happening. If this is only appearing within your department, but not the rest of the organization, it might be useful to look for your data group outside your department. #Labels anyone?
Wrapping up Retention and Cybersecurity Considerations
In most industries, at this point, you'll need to take a deep dive into written retention policies (if they don't exist, write one), and the type of data you have will dictate how strict of cybersecurity depth you need to consider.
For example, in medical facilities subject to HIPPA and the HIGH TECH Act, or credit-providing companies that have social security numbers there might be a different level of cybersecurity necessary than say, a spa that has pictures of you or your driver's license or FSA credit card on file (Flexible Spending Account for any non-US readers - yes our healthcare needs revamp). #Selfcare is critical!
In larger organizations, it's helpful if there's an internal audit department that you could work with to operationalize some of your data quality desires. I've found when there's someone outside the department with findings (even if those findings are just from Theo in Costa Rica), people often pay more attention to those requests. This is a tactic I've used sparingly, as it truly does only work in select circumstances.
Do you know your new system?
Oh, you thought we had already migrated did you? Well we're almost there. At this point, you really know the ins and outs of your list. Do you still even have it? Or has it morphed so much that it went from (hopefully!) caterpillar to butterfly? #neurospciy #ftw
If you've tended to your list while doing all these other things, then it should still be able to be rolled up and counted. Why counting you say? Well, often times when moving, small seemingly unnecessary things fall off the truck. A lot of these pathways this data is moving along can get bumpy, or get really cold and go underground. If you know your data, you should know what it's supposed to look like when it gets to the other side. Oh, did your chicken not make it to the other side? #roadkill
So the new system with it's roadkill entrails needs to look like a rockstar??
(#Rockadoodle fans anyone?) - though I've gotten new lenses (#intersectional) since last seeing it).
Migrate it over
The actual actual act of migration is fairly simplistic but beautifully complex. ETL, Extract, Transform, Load. Though, for most teams I've been on, you've ETL'd it to death. It killed your father, prepare to die (#PrincessBride). So it's likely just Extract and Load, but wait!
Yeah there is still transforms to be done on the new system. Unless you have total #greenspace where you're building something from scratch, there's often data limitations that you weren't aware of (think of wanting to store the letters A-Z, but the field only allows Numeric).
A bit outdated example as most data limitations are not formatting, but rather communication errors. Again, the more documentations the better.
Comments